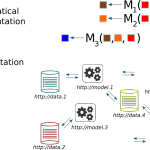
Towards the Semantic Description of Machine Learning Models
Semantic Web Technologies integrate naturally with the worlds of open data science and open source machine learning, empowering better control and management of the risks and opportunities that come with increased digitization and model use
The ongoing and accelerating digitisation of many aspects of social and economic life means the proliferation of data driven/data intermediated decisions and the reliance on quantitative models of various sorts (going under various hashtags such as machine learning, artificial intelligence, data science etc.).